In the previous articles, we discussed how bias creeps into deep learning systems from datasets and those biases are then amplified due to the very nature of these systems. In this article, we shall see what happens when these biased systems are put to test in the real world.
Zoom’s background problem
Zoom like many other videoconferencing systems offer the option of replacing the participants backgroundwith a virtual background. The system used is proprietary and so its workingis not available in public domain. The technology behind such systems generally uses deep learning. This technology has worked perfectly for millions of users connecting virtually during the pandemic, but it seems to have failed for some. And one such person happens to be unsurprisingly – black.
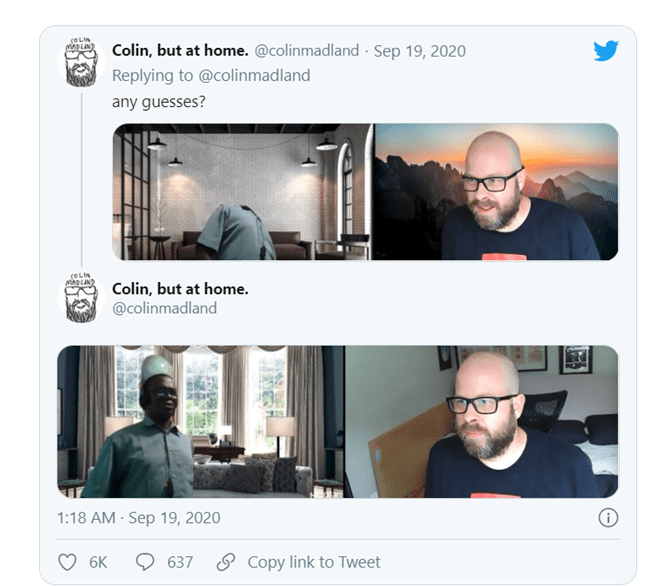
Zoom’s ‘vanishing act’. Source:TechCrunch[1]
As seen in the above picture, two people – one black and the other white – log into zoom and things go smoothly. But as the AI kicks in (with the virtual background), the black person’s face disappears. This means that the software failed to identify his face properly and mistook it for a part of the background. The same software worked fine for the white person[1].
White Obama?
Deep neural networks are increasingly being used for image and video upscaling tasks. Which means taking a low resolution grainy image or video and generating a higher resolution version of the same. This technology has found widespread usage in the video, entertainment and consumer electronics industry. But this technology has an unintended ‘whitening’ effect. When presented with images or videos of non-white people,the upscaled output is that of people with considerably lighter skin colour.
De-pixelation of a pixelated image offormer US President Barack Obama resulted in an image with lighter skin tone[2].
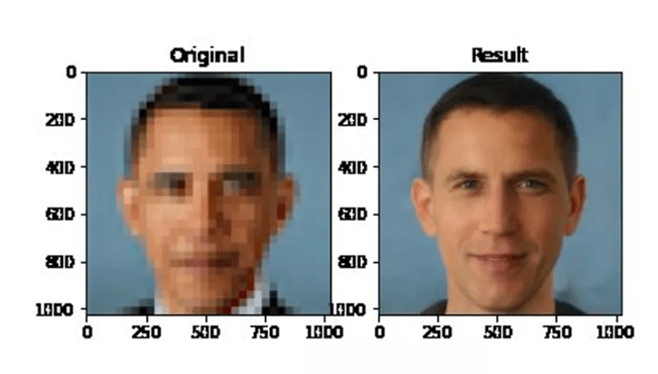
Racism ‘cropping up’ in Twitter’s algorithms
Twitter had introduced an image cropping facility for large, embedded images. When a large image is embedded in a tweet, an algorithm detects the ‘important’ parts of that image, which is then displayed embedded in the tweet. Some users checked it for racial bias and found that when presented with an image of black and white person, it picks the white person as ‘important’.
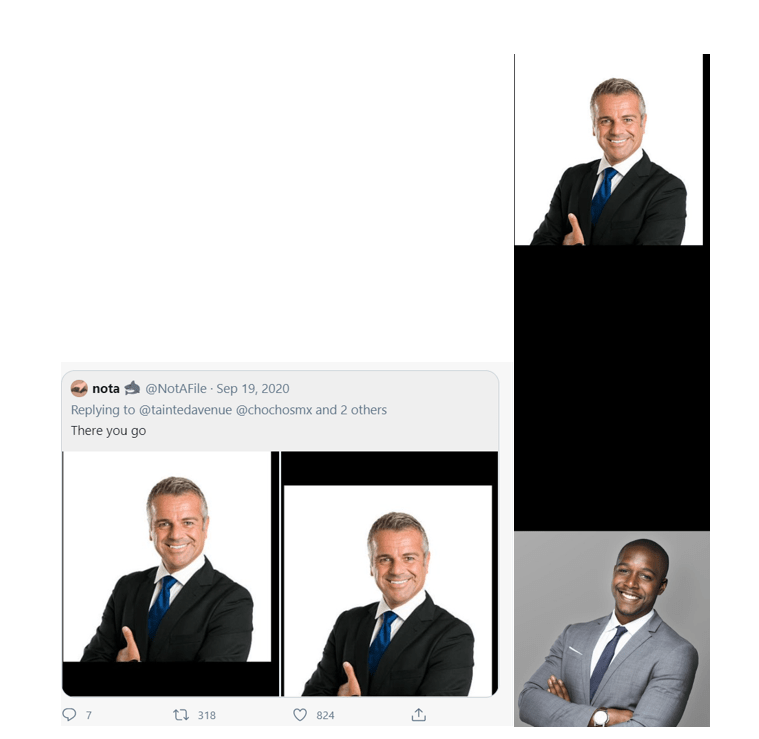
Cropped version of the image (left).Original image (right). Source: Tech Crunch[1]
The bigger picture
These issues are only the tip of an iceberg, a problem of titanic proportions – of systematic bias present in our society, data and AI. If unchecked, these issues can cause serious problems including loss of lives. From the examples above, it is clear that AI has difficulty inrecognising people of colour as humans. For a zoom virtual background, it means a bad video conferencing experience. But for a self-driving car, it can mean accidents; something that’s already happening[3].
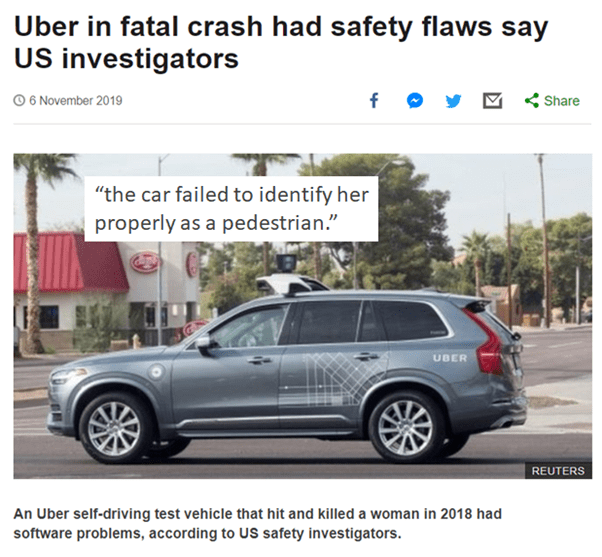
References
[1]M. Dickey, “https://techcrunch.com/2020/09/21/twitter-and-zoom-algorithmic-bias-issues/?guccounter=1”,Techcrunch.com, 2021. [Online]. Available:https://techcrunch.com/2020/09/21/twitter-and-zoom-algorithmic-bias-issues/?guccounter=1.[Accessed:31- May- 2021].
[2]J. Vincent, “What a machinelearning tool that turns Obama white can (and can’t) tell us about AIbias”, The Verge, 2021. [Online]. Available:https://www.theverge.com/21298762/face-depixelizer-ai-machine-learning-tool-pulse-stylegan-obama-bias.[Accessed: 31-May- 2021].
[3]”Uber in fatal crash hadsafety flaws say US investigators”, BBC News, 2021. [Online]. Available:https://www.bbc.com/news/business-50312340. [Accessed: 31- May- 2021].
Article 6 in a series by Abhishek Mandal, PhD Candidate University College Dublin + Dublin City University and Alliance / Women At The Table Tech Fellow
Last modified: February 27, 2022