The data we need for women’s health in the 21st century is missing.
The gender data gap is turbocharged by AI.
In the context of continuing and widespread AI adoption in healthcare, we run the serious risk of structurally embedding biases and gaps. Without being aware. Again.
AI learns from the data it’s trained on therefore if women are ‘invisible’ in that data, or misrepresented in the data this can have lethal consequences.
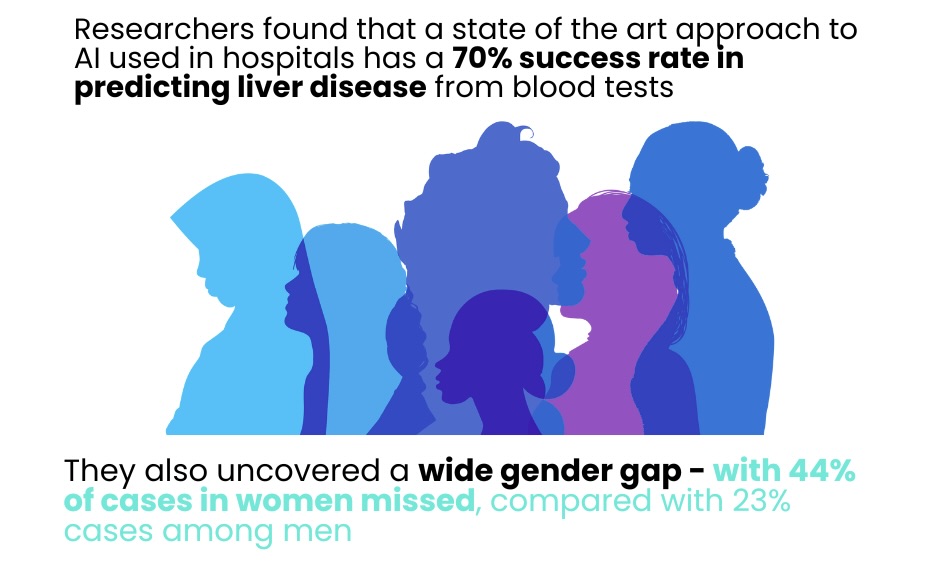
Gender bias revealed in AI tools screening for liver disease | Link
What is algorithmic bias in a healthcare context?
Defined for the first time in 2019 in the Journal of Global Health as “the instances when the application of an algorithm compounds existing inequities in socioeconomic status, race, ethnic background, religion, gender, disability or sexual orientation to amplify them and adversely impact inequities in health systems.”
We are becoming more and more aware of how this nexus of missing data sets and our speed in deploying AI models with missing data will entrench inequities.
How does bias enter into AI in health research?
Some examples of Types of Bias in Artificial Intelligence & their descriptions | Source
Bias | Description |
Inherent or Historical Bias | Even when data is accurately collected and sampled, models might yield undesired results due to pre-existing societal biases in the world. e.g. making the mistake of associating HIV primarily with gay and bisexual men because of its higher occurrence in this group |
Representation or Sampling Bias | When certain segments of the data input are not adequately represented. e.g. a large part of genomics research predominantly focuses on European male demographics, sidelining other ethnic groups. |
Data Proxy Bias | When the data we collect serves as an indirect measure for the desired attributes e.g. using various clinical, social, and cognitive indicators to identify early stages of schizophrenia, even though gender differences can influence the manifestation of these indicators and their related psychosis risk. |
Generalization/ Aggregation Bias | When a universal model is applied to groups that have distinct underlying conditions e.g. despite diabetes’ variable interpretations across different ethnicities and sexes. the widespread use of haemoglobin A1c (HbA1c) levels to diagnose and track the disease. |
Evaluation or Benchmarking Bias | When the data used to test or benchmark an algorithm isn’t a good match for the intended audience. e.g. the underwhelming performance of certain facial recognition technologies on individuals with darker skin tones, especially females, because most benchmark images are sourced from white males. |
Modeling Bias | Bias can be inadvertently or deliberately embedded into an algorithm, especially when relying on improvised solutions. e.g., when a commercial health prediction algorithm used healthcare costs as an indicator for health condition without factoring in prevalent disparities in healthcare accessibility, it displayed considerable racial prejudice changing predictions of genuine need. |
Synthetic Data
As we wait to collect the much needed missing data sets on women’s health there is a discussion about the use of synthetic data to augment data sets. This is a very promising approach on a number of levels but which has its own limitations if not thought through carefully.
Before using synthetic data to add to a dataset it must be noted that many patient cohorts had minimal participation in the original data. Statistics show that racial and ethnic minorities comprise 39% of the United States population but only account for 2% to 16% of clinical trial participants.
Factors like age, biological sex, disabilities, chronic comorbidities, geographical location, gender identity, race, and ethnic background may influence how an individual reacts to a certain drug, medical device, or treatment plan. If patients in clinical trials do not represent the whole community, there is the risk that differences in drug metabolism, side effect profiles, and outcomes will be missed.
This also translates when using synthetic data. The lack of diversity in synthetic patient cohorts can result in AI models that perform poorly on real-world populations.
As an example, generating data for 500 Black male patients and 500 Black female patients using a synthetic data generator trained on predominantly white medical records would not accurately reflect the true disease progression and outcomes experienced by Black patients.
To address this, representative real-world data must be collected first to ensure that AI models do not perpetuate healthcare disparities.
Moreover, the synthetic data landscape in healthcare is fraught with ethical considerations. While synthetic data offers the potential to accelerate medical research, drug development, and personalized treatment strategies, it must be used with care to avoid reinforcing biases and ensuring patient privacy and consent.
The opportunities for bias to enter into the data and machine learning lifecycle occur at every stage from inception, to System Requirement definition, data discovery, selecting and developing a model, testing and interpreting outcomes, and post-deployment/ Impact & Audit.
A multifaceted approach
Addressing gender data gaps in healthcare using AI requires a multifaceted approach, both in terms of technical solutions and systemic awareness. Here are some thoughts on a multi-dimensional strategy to fill these gaps, keeping the data limitations in mind:
- Acknowledge the Limitations: First and foremost, any AI solution should clearly communicate the limitations of the data it’s trained on. Users should be aware that predictions or insights may have inherent biases and are not likely to be as accurate for underrepresented groups, particularly women.
- Collaborate with Experts: Collaborate with gender researchers, sociologists, and clinicians who have expertise in women’s health. Their insights can guide data collection, feature engineering, and model evaluation.
- Crowdsource & Citizen Science: Engage the public, especially women, in collecting and contributing health data, including with wearables. Initiatives like Apple’s Research Kit have shown how valuable citizen-contributed data can be for medical research.
- Synthetic Data Augmentation: Using synthetic data augmentation techniques to artificially increase the size of underrepresented datasets. As noted above, this doesn’t replace real data, but it can help improve model performance by generating synthetic data based on existing patterns. With important caveats as ‘existing patterns’ can translate into perpetuating healthcare inequalities.
- Transfer Learning: Use models pre-trained on related tasks or datasets to benefit from their learned features. This can be particularly helpful if there are related areas of medicine where more diverse data is available.
- Meta-analysis and Data Synthesis: Conduct a meta-analysis of existing studies to derive broader insights. Even if individual studies are male-centric, combining results could help highlight patterns or trends that are relevant to women.
- Inclusive Model Development: Design models that explicitly account for gender and other demographic differences. For example, use multi-task learning where one task could be predicting a medical outcome, and another task could be predicting gender, making the model aware of gender differences.
- Regular Model Evaluation and Audit: Continuously evaluate and update the models as new data becomes available. This iterative approach ensures that AI solutions improve over time and remain relevant. If models ‘go rogue’ in the wild, retire them.
- Ethical Oversight: Establish an ethics committee or review board focused on AI in healthcare. This board can assess AI solutions for potential biases, ensuring that they meet ethical and inclusivity standards.
- Feedback Mechanisms: Implement feedback loops where clinicians and patients can provide input on AI predictions or insights. Over time, this can help in refining the model and making it more attuned to real-world nuances.
- Education and Training: Educate healthcare professionals on the limitations of AI tools, especially when they’re based on historically biased data. This education ensures that they can make informed decisions and remain critical of AI outputs.
- Push for Policy Change: Advocate for policies that ensure more inclusive and diverse data collection in future clinical trials and studies. Over time, this will help in reducing the data gaps.
By embracing these strategies, AI can be a powerful tool to fill the data gaps in women’s healthcare, while also ensuring that the solutions remain transparent, ethical, and continuously improve.
“If used carefully, this technology could improve performance in health care and potentially reduce inequities,” says MIT Assistant Professor Marzyeh Ghassemi. “But if we’re not actually careful, technology could worsen care.” (Source 1 | Source 2)
Last modified: May 22, 2024